Introduction
Over the last 10 years, there has been a huge explosion in the data created and retained by the companies. We name this massive amount of data as “Big Data”. Data science is a multidisciplinary union of technology, algorithm development, and data inference to solve the analytical problems which are complex.
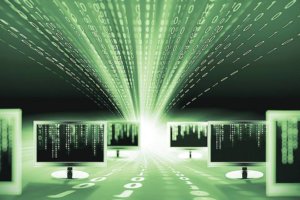
The core of data science is data. Stores of valuable raw data are transferred in bulk and accumulated in enterprise data warehouses. Data science utilizes innovative ways to ultimately create business value from this data:
Data Science – Discovery of Data Insight
Data scientists are the people who derive value from huge the massive raw data and make it useful to organizations. Data scientists start with an exploration of data to mine out data insights. They examine the leads and try to grasp the characteristics or pattern of the data.
Then, as needed, data scientists use creative techniques to explore at an in-depth level. The techniques include synthetic control experiments, forecasting, time series inferential models, segmentation analysis etc. The reason behind using these techniques is to observe scientifically what insights we are getting from data.
Data Science – Development of Data Product
A data product is defined as a technical asset that
- Uses data as an input
- Manipulates that data to return the results that are generated algorithmically.
This is different from the above section, the discovery of data insights, where the result of that is to render advice to an executive to make a wise decision related to his/her business. Data scientists play a key role in the development of data product.
This includes generating algorithms, testing, and technical deployment into the production systems. In this way, data scientists are functioning as technical developers and developing assets that can be utilized completely.
Data teams are creating robust, actionable analytics from massive data sets. These continuous attempts brought clarity to the people on how to communicate with the web and are the foundation for useful features that notify critical business approaches.
The demand for data scientists is increasing at a rapid pace that McKinsey estimates that by the end of 2018, there will be 50 percent gap of supply vs. demand for the role of data scientists. The major difference between data scientists and an engineer, or an analyst or a statistician is data scientist will perform each of those jobs.
The knowledge of classical as well as Bayesian statistics is required for a data scientist. When we talk about hacking here, we are not referring to breaking into computer systems. We are actually referring to the ingenuity and creativity in utilizing the technical skills to develop things and find the solutions to problems. Being a tactical business intelligence consultant is extremely important for a data scientist.
Possessing business acumen is as vital as possessing acumen for algorithms and tech. There needs to be proper alignment between business goals and data science projects. Ultimately, the value is not gained from tech, math, and data itself. It comes from making the maximum use of all the above to develop valuable capabilities and possess powerful business influence.
Conclusion
Data scientists are extremely valuable team members but are very rare in the talent market. This is due to the difficulty in acquiring their unique skill-set i.e., expertise in 3 areas as discussed above. The present demand for data scientists is sky-high due to the value they generate for the business. The median salary for a data scientist in the US is $112,000. People with training in data science are earning huge amount of salaries as stated earlier. If you are looking for an exponential career growth, you can choose data science as a career.
Savaram Ravindra was born and raised in Hyderabad, popularly known as the ‘City of Pearls’. He is presently working as a Content Contributor at Tekslate.com and Mindmajix.com. His previous professional experience includes Programmer Analyst at Cognizant Technology Solutions. He holds a Masters degree in Nanotechnology from VIT University. He can be contacted at savaramravindra4@gmail.com. Connect with him also on LinkedIn and Twitter.